News
How is technology actually helping?
[19.03.2025]Using learning data to gain actionable insights for students and teachers - that's what the Learning Analytics research professorship is all about. Since fall 2024, the team around CATALPA junior research professor Ioana Jivet has been complete with postdoc Kamila Misiejuk and doctoral student Volkan Yücepur. How do they want to support the learning process with their research? They explain in an interview.
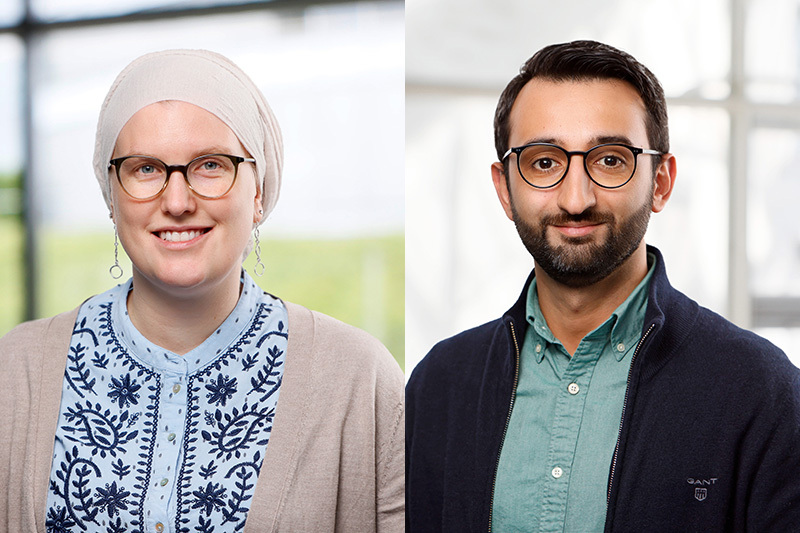
What are indicators
An indicator in the field of learning analytics is a measurable metric or key figure that provides information about the learning behavior and results of students. These indicators help to understand and analyze important aspects of the learning process.
For example, an indicator can show how often and for how long a student has attended online courses, how many exercises they have completed or how well they have performed in certain tasks. Such data is collected and evaluated in order to recognize patterns and draw conclusions about the student's learning success or possible difficulties and thus ultimately improve the learning process.
Kamila, Volkan, you are now well-rehearsed as a team. What are you working on right now?
I want to use learning analytics to help students make informed learning decisions. To do this, we must first identify the specific information needs of students. Then we consider how to translate those insights into learning analytics indicators. My goal is to be able to give teachers clear recommendations for use in their courses. There is still too little research in this area.
The information needs of students and the appropriate data sources are one of the three pillars of the research professorship in the development of tailored feedback systems. The second pillar is the provision and understanding of data, and the third is reflection and implementation by students. This structure really impressed me and was one of the reasons I applied to Ioana.
Kamila, which of these pillars are you focusing on?
Kamila Misiejuk: I deal with roughly three topics.
One is AI and how students are dealing with it and working with it. In other words, this new tool is now available and how do students use these tools for their tasks? So here I am more on the third pillar, which is student reflection and implementation.
My second focus is on peer assessment. This is where students assess each other's learning outcomes. At the moment I am particularly focused on what happens when AI is added to the peer assessment process. How does AI work in this complex system? Do students look at their peers' texts at all, or do they leave it alone because the AI is better anyway? How does it affect their motivation to look at the feedback if they have partially generated their texts with AI? With this work, we are moving between the second and third pillar, which is the understanding and subsequent implementation of feedback.
ENA and TNA
Epistemic Network Analysis (ENA)
ENA is a method for identifying and quantifying connections in data. It allows scientists to compare different networks visually and through summary statistics.
Transitional Network Analysis (TNA)
This method integrates a technique that visualizes processes using digital traces with a graphical representation of probability distributions. It is used to identify and visualize patterns of transitions and changes in learning process data.
The third topic I am working on is methods for data analysis, in particular network methods such as epistemic network analysis (ENA) and transition network analysis (TNA). I am particularly interested in how collaboration between students can be modeled. Basically, it is again about understanding and being able to represent complex processes. It's about data sources and how to make them useful to students or teachers, which brings us to the first pillar.
What fascinates you about these issues?
Volkan Yücepur: For one thing, I am fascinated by psychology. For example, I enrolled in the bachelor's program in psychology here in Hagen and was able to better understand the educational processes at the FernUni. At the same time, I am fascinated by computer science and all its possibilities. In computer science, we can not only digitalize existing processes, but also create completely new things. And with learning analytics in particular, we can quickly put research results into practice - unlike in disciplines such as medicine or biotechnology, where the transfer into practice can take much longer. And you Kamila? Why Learning Analytics of all things?
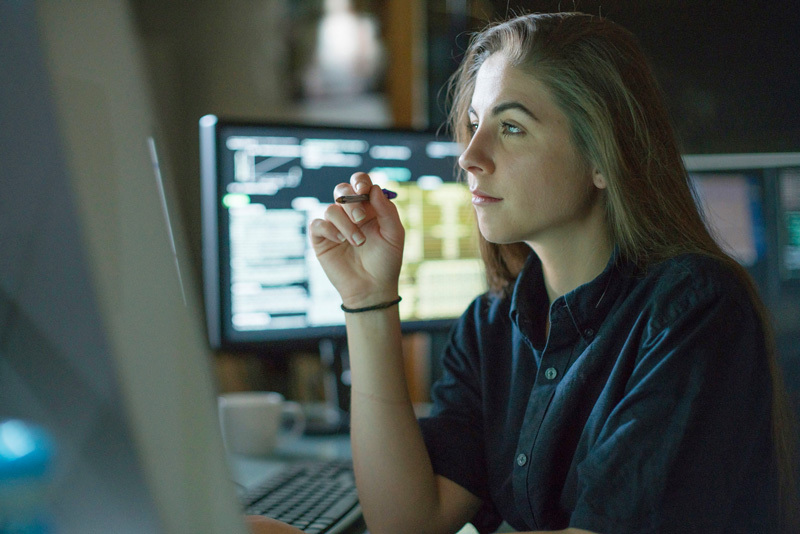
Kamila Misiejuk: When I started my Ph.D. in 2017, the world was still a bit different. It was before Covid and before the big breakthroughs in AI. Initially, I thought we could do anything with data. But it takes a lot of extra work to actually get useful insights that can improve educational practice.
With the emergence of educational technology and AI, new questions are being asked: In what areas are these technologies really helpful? Are there situations where they might even be harmful? Generative AI has revolutionized the entire field. The dynamics of the field require constant adaptation and reinvention.
Before CATALPA, what did the two of you do?
Kamila Misiejuk: I worked at the Center for the Science of Learning and Technology (SLATE) at the University of Bergen in Norway, where I completed my Ph.D. in 2023 with a focus on peer assessment and learning analytics.
During my time as a senior researcher, I have been involved in many different projects, all aimed at improving the learning experience through innovative technologies. One of my projects involved remote laboratories. This involved students not being physically present in the lab, but working from other locations. For this collaborative activity, I developed dashboards to help instructors and students.
Volkan Yücepur: I studied business informatics in Dortmund and was interested in the interface between software development and psychological aspects. In the master's program at the University of Duisburg-Essen, I was able to combine computer science and psychology, which also gave me an insight into learning analytics.
After graduating, I went into IT consulting to try out different opportunities. But then I saw the job at CATALPA, which couldn't have been more interesting for me personally. I remembered Ioana Jivet's name from the articles I used as sources for my master's thesis. Since the education sector was exactly where I originally wanted to work, this was the perfect opportunity.
Finally, I would like to look into the future with you. What do you want to achieve with your research?
Volkan Yücepur: My main goal is to come up with indicators and visualizations that teachers like to use and trust because they are scientifically proven. So far, there has been a strong focus on dashboards, similar to the structure you might see in corporate KPI visualizations. But these dashboards can be very complex, and not all students are good at understanding and interpreting data about their learning behavior to derive recommendations for themselves. The question now is how to provide students with the right information, at the right time, in the right place, without overwhelming them. Finding scientifically sound answers to this question is what drives me.
Kamila Misiejuk: In Scandinavia I had a strong network in the learning analytics community. I no longer have that here in Germany. That is one of my goals, to build up a stronger network here again. As a result of our science, I come more from the perspective of teachers. I think they have a strong influence and can use data-driven insights to make decisions that make a difference. For example, if they see that there are similar problems in certain areas, they can make adjustments to the course. So sometimes simple things can make a big difference. To achieve this, we need to have good, long-term cooperation with different teachers in the future. This is the only way we can do practical research, put the results into practice, and scientifically classify the effects of interventions.