News
FLEXI: Guidance on the local hosting of LLMs
[03.07.2024]How to deal with AI in university teaching? Which access points should universities provide for teachers and students? As part of the FernUni LLM Experimental Infrastructure (FLEXI) project, Torsten Zesch and an interdisciplinary team are dedicated to testing and using a locally hosted open Large Language Model (LLM).
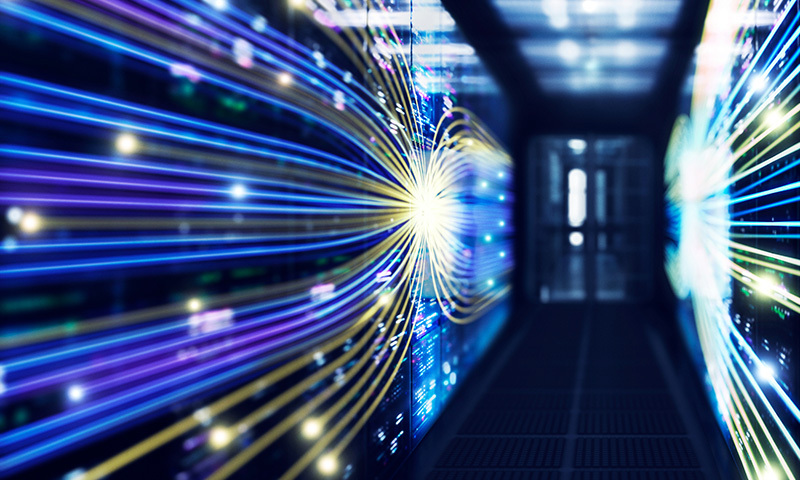
Hardly any evidence-based decisions to date
The potential that artificial intelligence in general and large language models in particular offer for higher education is huge. As long as access is not provided by the university in some way, everybody
is using the commercial service of their choice, leading to issues including potential data security
problems, decreased educational equity or potentially high costs. "The living lab approach of our research center allows us to test FLEXI in real-world applications," explained Torsten Zesch. And not just technically: CATALPA accompanies various application scenarios with research projects. "So far, there is hardly any evidence on the impact of large language models on teaching," says Zesch. The aim of FLEXI is therefore to provide urgently needed, scientifically sound findings for or against the use of these local solutions.
Cloud vs. local
For the experimental environment within FLEXI, the researchers had the choice between a locally hosted open LLM or the cloud-based variant. "Cloud-based systems are easy to set up and ensure that the latest models can be used. Open LLMs are currently more cost-effective and data protection is much easier to ensure. For these reasons, we opted for the open LLM at FernUni," explains Zesch. FLEXI is intended to enable experimentation with a locally hosted, open Large Language Model, both in teaching and in science.
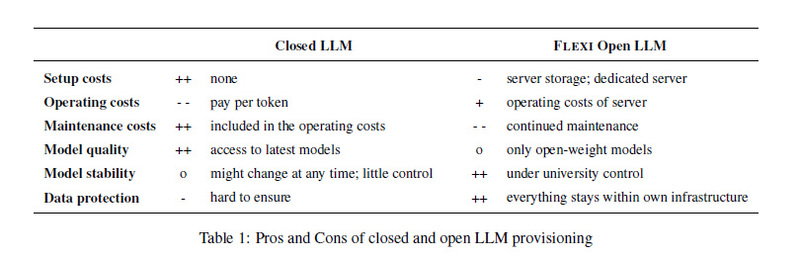
Practical guidance
In a new publication, CATALPA members Torsten Zesch, Michael Hanses, Niels Seidel, Claudia de Witt and Dirk Veiel some practical guidance for everyone trying to decide whether to run their own LLM
server. At the time the paper was published, there were 90 different models to choose from - all with their own strengths and weaknesses. In the paper, Zesch and his co-authors show which criteria they used to select the models, which quality tests ran and which use cases they are currently developing.
"We must not only support the higher education landscape in creating access to AI and LLMs, but also critically question how the technology actually offers added value for learning and teaching," concludes Torsten Zesch.