News
Computational Linguistics: About Orthographic Errors and Effective Arguments
[31.07.2023]Automatic scoring and handwriting recognition in learners' freely written texts - these are the topics on which four papers from the CATALPA Research Professorship in Computational Linguistics and the EduNLP Junior Research Group were accepted at ACL 2023 in Toronto. The "Annual Meeting of the Association for Computational Linguistics" is one of the most important conferences for researchers in this field.
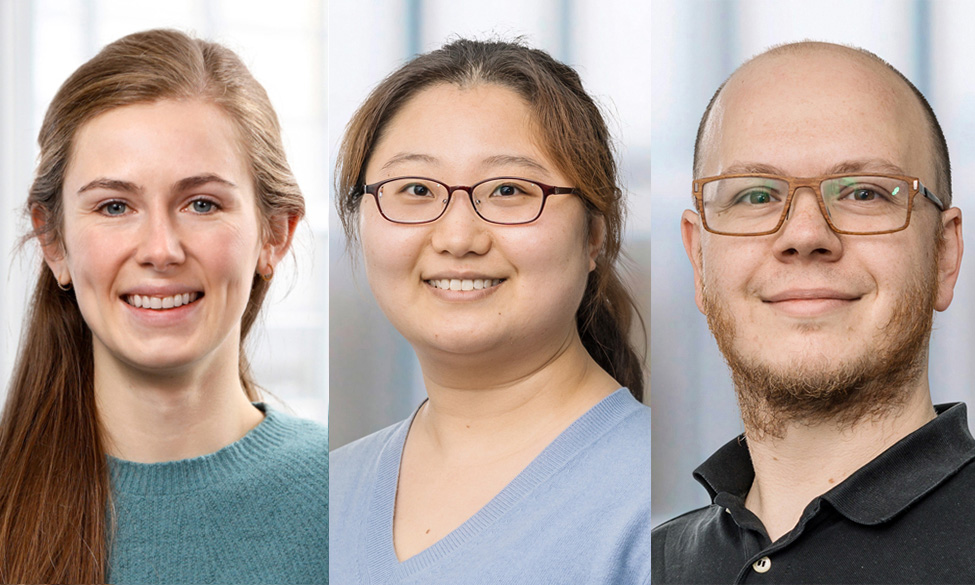
Science communicator Christina Lüdeke asked Marie Bexte, Yuning Ding and Christian Gold about their research findings.
Christina Lüdeke: Christian, you are working on handwriting. What exactly is the focus of your research?
Christian Gold: It's about handwritten texts with errors. Learners make orthographic errors when they compose texts. Or they strike through words and write others over them. Sometimes they also make an asterisk and insert new words, which they write down in a different place of the page. When I have a camera capture all of this, it's just pixels of color information for now. My goal was to use AI to capture everything written letter by letter - and with the spelling mistakes that the learners made.
Christina Lüdeke: What do you expect from this?
Christian Gold: Many learners have difficulties with certain spelling rules, and some also have dyslexia. If handwriting is recognized automatically, such errors and weaknesses can also be identified early on. Teachers can then provide targeted support. But there has been very little research in this area so far. I have done some foundational work here, so to speak.
Christina Lüdeke: But the topic of automatic handwriting recognition is not new...
Christian Gold: Yes, but most of the time other researchers are concerned with automatic recognition of historical texts, for example church records. And even if the texts are current, errors are always normalized away during the automatic recognition process, because it's usually about the content. That was different in our project, where the focus was on the orthographic errors.
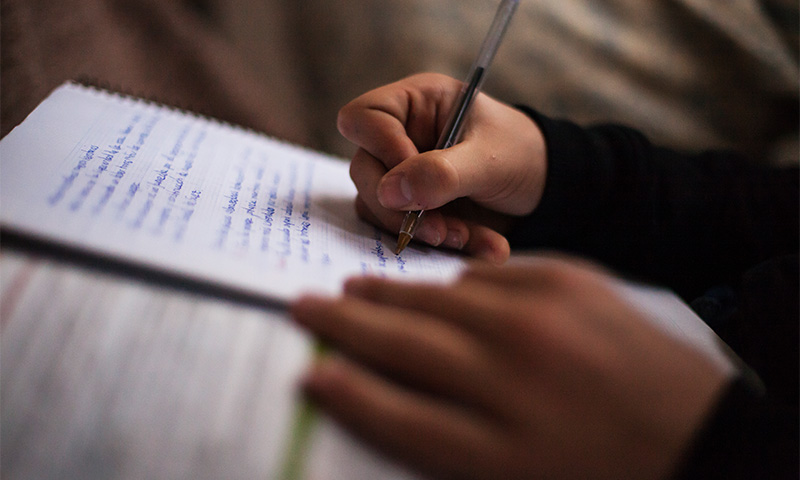
Christina Lüdeke: How did you proceed?
Christian Gold: The University of Cologne had already transcribed a large number of freely written texts for another research project. However, the focus was on content, not on spelling. That's why we started the transcription process all over again: Two annotators re-transcribed 1,350 texts according to specific guidelines. For example, the letters 'd' and 't' can be mixed up in the German word 'Wald'. Then we generated a kind of dictionary for orthographic errors. We assumed a vocabulary of 45,000 words, matching the learning level in our group. But when you include a large number of possible errors, the dataset gets very large very quickly. Our dictionary of orthographic errors then included about 14 million variants of these words, because there are so many ways to misspell something.
Christina Lüdeke: Wow, that sounds like a lot of work.
Christian Gold: Yes, it was, but it really allowed us to capture almost all of the misspelled and correctly spelled words in the manuscripts, a total of 94 percent. Because the transcription process alone was so challenging, we made two papers out of our work. It would have been beyond the scope of one. Other researchers working on a similar topic can now build on this foundation.
Essays and their evaluation
Christina Lüdeke: You two, Marie and Yuning, are also looking at learner texts. But you're not looking at spelling, you're looking at content, right?
Yuning Ding: Yes, exactly. My paper is about argumentative essays and their evaluation, i.e., automatic scoring. For example, I myself studied English as a foreign language, and I always found free writing the most difficult. But this is also where it is most burdensome for teachers to provide feedback. Their resources are simply limited. This is where automation can make an important contribution in the future to support learners.
Christina Lüdeke: How did you go about it?
Yuning Ding: On the one hand, we were concerned with the structure of the texts. An argumentative essay consists of, among other things, a lead, claims, evidence for the claims, and a conclusion at the end. That was the first challenge, that these segments were clearly recognized and assigned.
Christina Lüdeke: And what else was it about?
Yuning Ding: First and foremost, an evaluation of the content, of the effectiveness of the argumentation. Assessing this is central to the evaluation of the text as a whole.
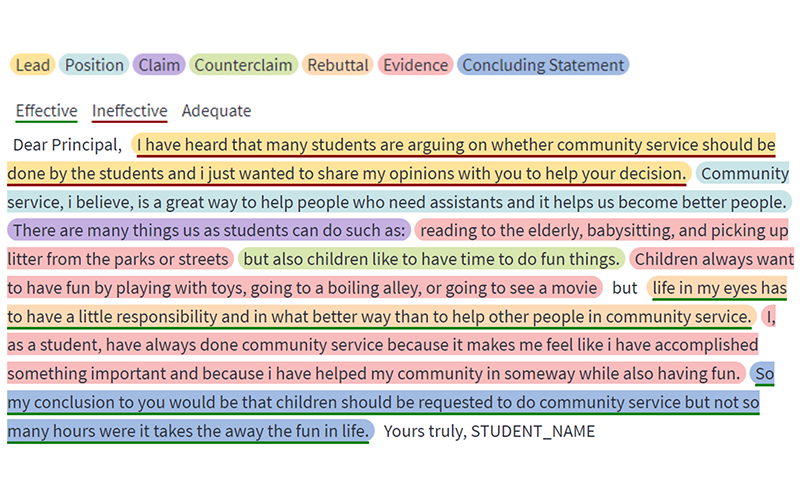
Christina Lüdeke: And this effectiveness can actually be evaluated automatically?
Yuning Ding: Yes, it works quite well, especially if we include the writing prompt for the learners. For the text dataset that we used, the teacher's evaluation is also available in each case. That's our gold standard, so to speak. We compared our automatic scoring with this. It works particularly well when we jointly learn the segmentation and classification of the texts and the scoring of the effectiveness. This brings us closer to the teacher's evaluation than if we consider the two separately.
A comparison of scoring methods
Christina Lüdeke: In your work, Marie, you compare different scoring methods with each other - "instance-based scoring" and "similarity-based scoring." Which is which?
Marie Bexte: The usual method when it comes to scoring freely written texts is "instance-based scoring". For that, you need very large datasets. The AI compares the texts with each other and determines an evaluation, i.e., the score, but without humans being able to understand exactly how this score is arrived at. With similarity-based scoring, on the other hand, a model solution is provided, so to speak, with which the texts are then compared.
Christina Lüdeke: That is closer to the approach of a human teacher...
Marie Bexte: Yes, and you don't need as much data as with instance-based scoring. Usually, I don't have thousands of people in a learning group working on the same task. Therefore, the method is much closer to the realistic learning setup. The teacher can exert more influence on the score because he or she sets the standard. In addition, we assume that the learners' acceptance of the score is higher if they can understand how it was arrived at.
Christina Lüdeke: Sounds like the method has many advantages. So how did it compare?
Marie Bexte: From a previous study, we had indications that both procedures could perform equally well. We have now examined this more closely here and found that both methods each have their strengths and weaknesses. Nevertheless, the advantages of "similarity-based scoring" remain, of course. Therefore, we next want to investigate a hybrid approach that combines both methods.
Conference as a milestone
Christina Lüdeke: You will be going to Toronto to present your paper and also Yuning's paper, for which you are a co-author. What does that mean for you?
Marie Bexte: To be at such a top conference with my own long paper - that is already an important step in my scientific career. I am really looking forward to it.
Yuning Ding: For me, the work that went into the paper was also an important milestone. Unfortunately, I can't go to the conference because I'm going on a research stay in the USA. But I've done maybe a third of the work for my dissertation, which means a lot to me.
Christina Lüdeke: And what about you, Christian?
Christian Gold: I have completed the research part of my dissertation with this work. Now I "only" have to write it down. Therefore, the conference is clearly a milestone for me as well.